Outrider is using advanced models in a hybrid cloud to improve throughput in automated truck yard management.
The post Outrider uses reinforcement learning to speed path planning by tenfold appeared first on The Robot Report.
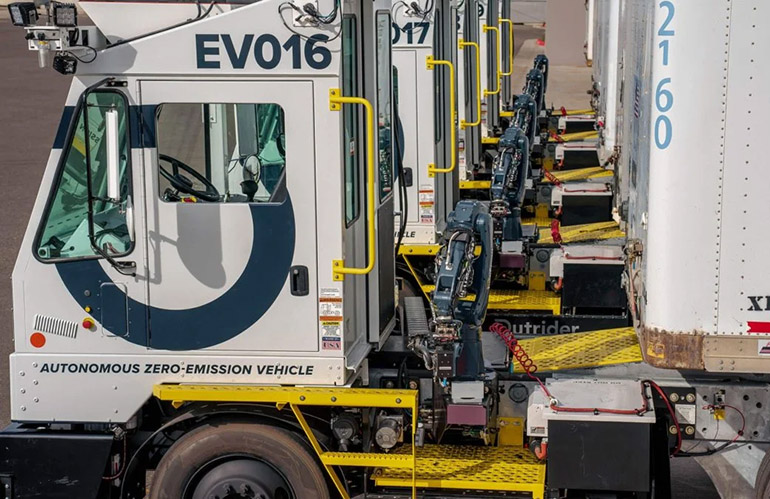
Outrider is using reinforcement learning to enhance throughput at truck yards. Source: Outrider
Outrider Technologies Inc. today said it has deployed advanced reinforcement learning, or RL, techniques to maximize freight throughput at customer sites. The company said its RL models can increase path-planning speed by 10x and enable the Outrider System to move freight more efficiently and safely through busy, complex distribution yards.
“Using the latest advances in AI, Outrider is continually decreasing the turn time of trailers moved autonomously in logistics yards,” said Vittorio Ziparo, chief technology officer and executive vice president of engineering. “By training and evaluating our system performance with RL in simulation and real-world scenarios, our customers see incremental improvements in speed and efficiency with our technology.”
Outrider is focused on automating yard operations for logistics hubs to help large enterprises improve safety and increase efficiency. The Brighton, Colo.-based company said it works with enterprises to eliminate hazardous and repetitive manual tasks.
Reinforcement learning to improve yard efficiency
Enterprises in package shipping, e-commerce and retail, consumer packaged goods, and manufacturing are looking to automate manual tasks in logistics yards to increase efficiency and improve safety. By using reinforcement learning, Outrider claimed that it enables logistics customers to realize the benefits of artificial intelligence in the physical world more quickly.
“Our partnerships with priority customers are facilitating these major industry advancements,” added Ziparo.
Outrider said its AI-driven capabilities are complemented by redundant safety mechanisms, combining the benefits of AI with traditional functional safety approaches used for industrial operations. The company said it has addressed more than 200,000 safety scenarios, and multiple third-party safety experts and Fortune 500 customers have validated its safety case.
RL techniques involve creating a model that improves decision-making over time.
Using years of data samples of behaviors, Outrider developed an RL curriculum of increasing difficulty for the model to learn. This technique reinforces preferred behaviors, such as following traffic rules and maintaining safe distances from other vehicles, and discourages undesirable behaviors.
Once the RL models are tested extensively in simulation and on-vehicle at Outrider’s Advanced Testing Facility, the model and code are deployed into autonomous operations at customer sites.
“Our Fortune 500 customers’ yards are complex, with hundreds of trucks, trailers, other vehicles, and pedestrians operating onsite daily,” added Ziparo. “RL is critical to automating these yards at scale because it enables our commercial system to handle increasingly complex and diverse environments – from distribution and manufacturing yards to intermodal and port terminals.”
The company has deployed zero-emission systems to drive adoption of sustainable freight transportation. “Outrider is the first-to-market yard automation solution that performs fully autonomous, zero-emission trailer moves,” it said.
Outrider uses models in hybrid cloud
Outrider’s reinforcement learning techniques use millions of proprietary, yard-specific data points collected and labeled across various large, complex distribution yards in multiple industries. These data points feed Outrider’s proprietary deep learning (DL) and RL models to create neural networks that automate yard tasks with increasing intelligence, precision, and speed.
Processing these data points through DL and RL models requires sophisticated computing hardware and a cost-effective training environment on a hybrid of public and private AI clouds. Outrider’s private AI cloud deployment uses NVIDIA’s DGX H200 graphics processing units (GPUs) installed at a secure, Denver-based data center owned and operated by Equinix.
“When dealing with exponentially increasing amounts of data to train DL and RL models, processing speed and training velocity per dollar spent matters,” said Tom Baroch, senior director of global partnerships at Outrider.
“NVIDIA, an investor in Outrider, helped us secure the cutting-edge hardware necessary to double our DL training speed and we deployed the hybrid cloud training environment, which increased training velocity per dollar by six times,” he said. “Taking this approach, Outrider delivers even greater value sooner to our customers.”
The company said RL facilitates its fully autonomous trailer moves, including hitching, backing, trailer brake-line connection, yard inventory tracking, and integration with warehouse, yard, and transportation management systems.
The company said its deployment of RL models bookends a year full of accomplishments. Highlights of 2024 included securing multiple patent grants and raising $62 million in Series D funding.
The post Outrider uses reinforcement learning to speed path planning by tenfold appeared first on The Robot Report.
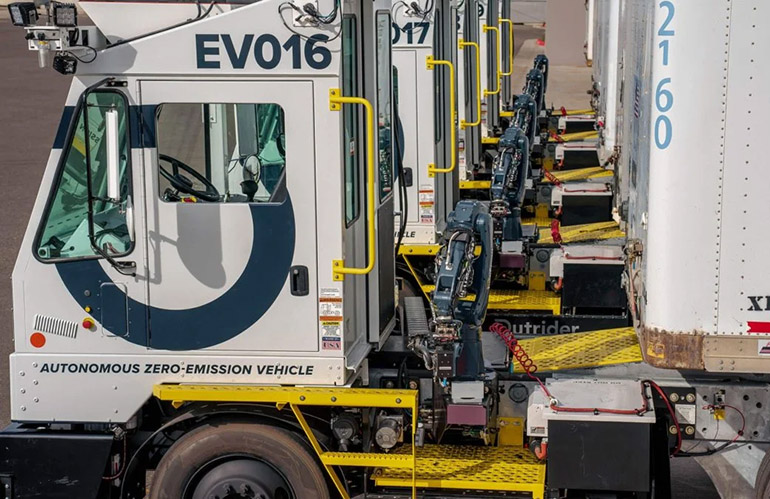
Outrider is using reinforcement learning to enhance throughput at truck yards. Source: Outrider
Outrider Technologies Inc. today said it has deployed advanced reinforcement learning, or RL, techniques to maximize freight throughput at customer sites. The company said its RL models can increase path-planning speed by 10x and enable the Outrider System to move freight more efficiently and safely through busy, complex distribution yards.
“Using the latest advances in AI, Outrider is continually decreasing the turn time of trailers moved autonomously in logistics yards,” said Vittorio Ziparo, chief technology officer and executive vice president of engineering. “By training and evaluating our system performance with RL in simulation and real-world scenarios, our customers see incremental improvements in speed and efficiency with our technology.”
Outrider is focused on automating yard operations for logistics hubs to help large enterprises improve safety and increase efficiency. The Brighton, Colo.-based company said it works with enterprises to eliminate hazardous and repetitive manual tasks.
Reinforcement learning to improve yard efficiency
Enterprises in package shipping, e-commerce and retail, consumer packaged goods, and manufacturing are looking to automate manual tasks in logistics yards to increase efficiency and improve safety. By using reinforcement learning, Outrider claimed that it enables logistics customers to realize the benefits of artificial intelligence in the physical world more quickly.
“Our partnerships with priority customers are facilitating these major industry advancements,” added Ziparo.
Outrider said its AI-driven capabilities are complemented by redundant safety mechanisms, combining the benefits of AI with traditional functional safety approaches used for industrial operations. The company said it has addressed more than 200,000 safety scenarios, and multiple third-party safety experts and Fortune 500 customers have validated its safety case.
RL techniques involve creating a model that improves decision-making over time.
Using years of data samples of behaviors, Outrider developed an RL curriculum of increasing difficulty for the model to learn. This technique reinforces preferred behaviors, such as following traffic rules and maintaining safe distances from other vehicles, and discourages undesirable behaviors.
Once the RL models are tested extensively in simulation and on-vehicle at Outrider’s Advanced Testing Facility, the model and code are deployed into autonomous operations at customer sites.
“Our Fortune 500 customers’ yards are complex, with hundreds of trucks, trailers, other vehicles, and pedestrians operating onsite daily,” added Ziparo. “RL is critical to automating these yards at scale because it enables our commercial system to handle increasingly complex and diverse environments – from distribution and manufacturing yards to intermodal and port terminals.”
The company has deployed zero-emission systems to drive adoption of sustainable freight transportation. “Outrider is the first-to-market yard automation solution that performs fully autonomous, zero-emission trailer moves,” it said.
Outrider uses models in hybrid cloud
Outrider’s reinforcement learning techniques use millions of proprietary, yard-specific data points collected and labeled across various large, complex distribution yards in multiple industries. These data points feed Outrider’s proprietary deep learning (DL) and RL models to create neural networks that automate yard tasks with increasing intelligence, precision, and speed.
Processing these data points through DL and RL models requires sophisticated computing hardware and a cost-effective training environment on a hybrid of public and private AI clouds. Outrider’s private AI cloud deployment uses NVIDIA’s DGX H200 graphics processing units (GPUs) installed at a secure, Denver-based data center owned and operated by Equinix.
“When dealing with exponentially increasing amounts of data to train DL and RL models, processing speed and training velocity per dollar spent matters,” said Tom Baroch, senior director of global partnerships at Outrider.
“NVIDIA, an investor in Outrider, helped us secure the cutting-edge hardware necessary to double our DL training speed and we deployed the hybrid cloud training environment, which increased training velocity per dollar by six times,” he said. “Taking this approach, Outrider delivers even greater value sooner to our customers.”
The company said RL facilitates its fully autonomous trailer moves, including hitching, backing, trailer brake-line connection, yard inventory tracking, and integration with warehouse, yard, and transportation management systems.
The company said its deployment of RL models bookends a year full of accomplishments. Highlights of 2024 included securing multiple patent grants and raising $62 million in Series D funding.
The post Outrider uses reinforcement learning to speed path planning by tenfold appeared first on The Robot Report.